Notes from FIMA London 2022, one of the leading financial data conventions.
15 November 2022
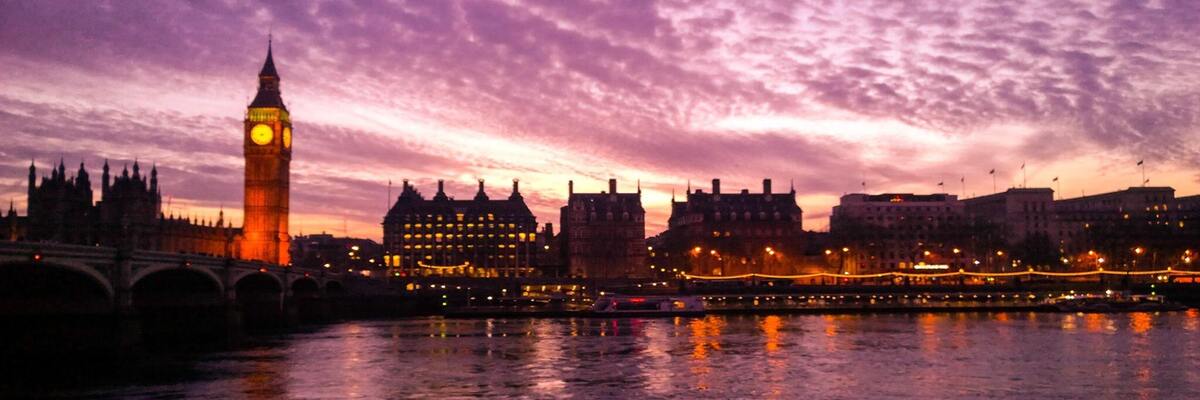
FIMA London 2022
My notes are not covering the full conference as this conference has several parallel tracks going on.
How can you adapt your data capabilities to meet ever-increasing ESG Data Demands and Business-critical sustainability goals?
Caroline Blaimont, Data Governance and Head of ESG Data program, BNP Paribas Fortis
Charmaine Wong, Group Head of ESG Data, HSBC
Chris Pim, Head of Master Data Management, Natwest
John Bottega, President, Enterprise Data Management Counci
Personal note: I came in while the panel was already going on, so my notes are incomplete.
The polling question reveals that only about half of the organizations have a dedicated ESG data program. It is somewhat surprising that 25% has not even started yet with a data program focused on ESG data. Another polling question reveals that only 6% of data teams are ultimately responsible for ESG reporting and only 19% are very involved. 20% is not involved at all.
There are a lot of challenges with the definition of what is ESG-compliant. What is an oil company that massively invests in solar energy?
Sometimes relationships with clients just have to be stopped, if it is noted that the direction is not the right one. The interpretation of the scoring of external parties is quite hard and not at all aligned. Different external parties give different scorings, which makes it hard for the financial institutions to make evaluations. There is a need for more transparency on the way that scoring is performed.
Questions to ask are: have we defined our data consistently? The answer is clearly no. Do we have a trusted source for ESG data? Not at all.
Different taxonomies are popping up, which will provide an additional challenge to banks that work across borders. Currently, there are more than 18 major taxonomies. All systems that are being built, will need to be flexible.
Currently, there is so much uncertainty on how to tie all these data together.
Organizations will need to set their own North Star today, knowing that standards will evolve. You cannot remain immobilized and wait around until the final standard comes in.
Upskilling will be very much required because this field requires skills in data management, financial management, risk management and environmental science.
Do we believe that we deal in the correct order with ESG? Should we not treat the Social dimensions first, so that the Environmental issues can get resolved in a sustainable and socially responsible way? From a data perspective, the biggest urgency is on the environmental data.
Personal note: to my surprise someone in the audience questions the impact of human activity on global warming. No further comment.
There is an agreement that this industry cannot afford to fail in this field.
How to Transform Your Data Organisation into a Powerhouse to Enable Faster Analytics and Enhanced
Decision Making
Rakshit Kapoor, Chief Data Officer UK and Head if Data Transformation Europe
Helena Schwenk, VP, Exasol
In terms of reporting, the audience poll shows that 44% of CDO’s report to COO or CFO. Only 10% reports to the CEO. 33% reports to a technology executive.
Public research points to the fact that CDO’s that connect well with the business side of the organization are the most successful.
Getting the buy-in of the board is a key-success factor. So much in the financial industry involves data. So therefore the board needs to be deeply educated.
There is no real moment when we will be “done” with data.
Finding out the board metrics is crucial. This could be about customer growth, ESG, diversity and inclusion, cost, etc. It is critical to be able to connect the activities of the CDO to the key metrics. It means that priorities for the CDO need to be tied to and prioritized along the business priorities. On the other hand, the CDO needs to be firm on insisting that certain foundations are being built.
Regardless of the debate about centralization or decentralization, it is impossible to operate with champions close to the business. The level of centralization can also depend on temporary situations. In case of an urgency, centralization may be required. In a non-urgent, non-cost sensitive context, decentralization might be the more agile solution. So you always end up in a hybrid approach.
Inside a long term plan, it is important to assign intermediate milestones to which a board can relate and see progress. Data people need to communicate in terms of data outcomes, not in terms of their own data language.
Various factors influence the tenure of the CDO. It is a hard job, with many stakeholders and a lot of varying and sometimes conflicting priorities. Also, the market for CDO’s is hot, so CDO’s are moving around, if not for better compensation. It may take another decade before the role becomes stable.
CDO’s need to manage how much burden they self-create that will hinder time-to-market for data products.
How can you embed ESG into your data strategy, comply with incoming regulations and drive sustainability?
Simone Steel, Chief Data and AI Officer, Nationwide
Rakshit Kapoor, Chief Data Officer, Santander UK
Martina Macpherson, Head of ESG Product Management Financial Information, SIX
Gurprit Singh, Global head of Data, Partners Capital
What is the difference between the ESG field and the good old corporate social responsibility? Strangely, no company seems to be disclosing bad ESG indicators.
You could draw the parallel with GDPR. Privacy had always been a concern, but the appearance of a stick from regulation, focusses the attention. The same is happening with the ESG topic. Banks, asset managers, insurance companies can play a very prominent role in the required environmental, social and governance reforms that the world needs.
Various reportings and disclosures now move from voluntary disclosure to mandatory disclosure. The financial rating suppliers are adding ESG data to their datasets but at the same time a lot of specialized data providers pop-up. At the same time, a lot of frameworks and taxonomies come into life.
Asset management clients are aggressively looking for ESG positive investment products.
A consistent data management framework was already important for other reasons. The same principles apply for ESG data and ESG data management. However, there are gaps in the availability of data sets. A lot of the reporting already exists but there is a growing number of stakeholders so related data needs to be repackaged for different stakeholders and regulators.
The UN Nations have assessed that there are more than 800 regulatory frameworks for ESG, pointing to the enormous confusion in the field.
ESG ratings only correlate about 60% between different rating agencies, while that is 99% for traditional credit rating. So as an organization, you need to understand what the rater is exactly rating and whether that fits for you.
Personal note: Happy to hear someone really ask this question of relevance, and not just preach for more and more and more data.
The ESG scene is ballooning out of proportion because the effort is no longer proportional to the resulting change of behavior.
In the polling question, the question is raised to which extent organizations are aware of their IT carbon footprint. 43% says "not at all", with only 20% being fully aware.
We keep adding layers of complexities in data storage and data processing and that should become a concern as well. Studies show that 80% of the information that we generate is never re-used.
In terms of managing external data sources, many organizations are in an infancy stage. There are challenges with the integrity and the quality of the data, with no verification mechanisms. Data quality concerns are the most prevalent issue. We are not short of data, we do not have the right data. There is a lot of analyst work involved in creating correct data. Such data creation and curation is expensive, which means that the use-case for ESG data must be examined more carefully.
The procurement process can play an important role in aligning a financial institution to become ESG aware.
How to leverage cloud-enabled storage and build data intensive applications without operational burden so you can scale with less risk.
Rinesh Patel, Global Head of Industry, Financial Services, Snowflake
The financial industry is undergoing structural change, technological change and commercial change. Major trends are the pressure on profitability, rising regulatory pressures, new entrants, changing customer demographics, sustainability and ethical investing, need for new revenue streams.
New business challenges are limited access to the view on the customer, delayed insights, inability to incorporate ESG metrics, costly and inefficient regulatory reporting, delayed insights to fight financial crime and a constrained customer experience.
Snowflake focusses on sourcing, aggregating, querying and sharing data.
Data sharing is more effective than moving large amounts of data. Organizations can build new applications on the platform.
How to govern and utilize your data to create a competitive advantage whilst complying with all your regulatory requirements
Tony Beals, Head of Data Governance, Natwest
Are competitive advantage and regulatory compliance opposing each other? Both impact cost/income, risk and growth.
76% of people in the room consider that there is indeed a clash between regulatory compliance and competitive advantage.
Personal note: I believe regulatory compliance is part of competitive advantage, not an opposing force.
The concept of “polarity” can is not really helping organization. It is not one versus the other.
Companies spend a lot on “change” and there never seems to be enough money. Still, the absolute amounts of money are high.
Asking the 5 why questions can help.
It can be very helpful to show how a particular use case contributes both to regulatory compliance as well as competitive advantage.
The 10 commandments for creating transformational customer outcomes through relentless data innovation
Graham Smith, Natwest Group
Key tensions to true data innovation are endless experimentation, legacy tech and lack of engagement. It requires a focus on value, a long-term technological capability and taking others along the journey.
1. Earn the right
It takes quite some time to build long term transformational initiatives. Organizations often start with foundational, basic applications that are not very complex (e.g. robotics, segmentation,...) . They grow towards more impactful applications, requiring more complexity.
2. Balance innovation pillars
Balance between new technologies, methods and data or finding business value. Push up on the three pillars at the same time.
3. Structure liberates
If you want to move from an idea to real value, a lot of things need to happen. Make sure not to have to re-invent this industrialization approach each time.
4. Build for scale
Make sure you can build and scale through time. Have a "feature-bank" and keep them up to date.
5. Developer experience matter
Care for the specialised talent. Try to make engineering processes good enough to retain the talent of today and tomorrow. Focus on ability to get things live. Communities of practice help to create a sense of belonging.
6. Nurture research freedom
If you want to keep people engaged, they need to be able to explore. This might entail the application of existing IP or the creation of new IP. Applications are vulnerability detection, false positive reduction, anomaly detection, credit risk protection, explainability, agent-based modelling, graphs features, pricing, conversation intelligence, customer life value, etc.
7. Create data products, not solutions
Create look and feel of product, communicate about it, think like a business, compete with the market.
8. Data innovation is a team sport
Elements of a team consist of business product owners, data scientists, data engineering,...
9. Innovate your governance and controls
Make sure that governance is supported by automation as well.
10. Learn to tell stories
All the other elements are not relevant if you cannot pitch your achievements. It's hard for data people to tell the story to non-data people. What matters is what the person at the other end is thinking about. Only 25% of the conversation should be about data, the other 75% should be about what matters to the business people.
How to collaborate with the business to develop a blueprint for data marketplaces which Gives Your Enterprise Access to Relevant Data Faster
Stan Reeve, Head of Data Marketplace, Legal and General Investment Management
Stuart Toll, Lead Architect, Data Platforms, Legal and General Investment Management
Elements of data strategy include Discovery, Access, Integrity, Accountability, Insights.
An architecture consists of various data loaders, a data store, a data store front and a studio, governed by a data control center.
The approach that was taken consists of the identification of business data challenges, have design principles, have platform requirements, have tech design principles, shortlist platforms, have vendor presentations, all under working hypotheses and recommendations. A cloud first strategy was adopted to provide the required agility.
The principle of a data mesh architecture was adopted, under the principle "Centralize the Core, Distribute the Value". The central data store holds a common data model, integration capabilities and a catalog, data quality control and data control. An "integration-as-a-service"-bus was implemented to facilitate ingestion. The pattern is more that of ELT. Raw data is loaded and the central team processes and refines and transforms data towards a common information model. Data quality checks are overlaid.
By enabling the distributed business teams with a storefront and a data studio, the central team does not become a bottleneck.
Applications include: Transparency of risk profiles of funds, profitability analysis, ESG score generation, creation of ESG exclusion lists,...
Data control is essential to guarantee data reliability and integrity. Federating consumption across the business, allows for cost management by avoiding a cost explosion centrally. Client and business value should direct all investments and work. Business must lead and technology and data must enable.
Innovation Use Case – Unlock the Full Potential of Your Data Mesh/Data Fabric Using Contextual Decision Intelligence Techniques
Imam Hoque, CEO and Founder, Quantexa
The first polling question suggests that 48% of people present have not formalised their data strategy and remain fragmented.
Personal note: This question defined "data strategy" more as "data technology strategy".
Classical areas in banks include customer intelligence, KYC, Credit Risk, AML and Fraud. Each of these areas run through a cycle of onboarding, on-going review and off-boarding. For each of these, various decisions need to be taken and these are contextual.
Contextual Decision Intelligence can help unlock the power of data and analytics as it goes further than just putting data in front of people with business intelligence.
"The network" is essential to understand the context of what role entities play in a certain dynamic. This can reduce false positives in AML, boost referral sales in a b2b context, insurance claims validation, etc.
The second polling reveals that the major roadblock would be the data quality and the availability of resources and skills.
Key components are entity resolution, network generation, advanced analytics and visualization/exploration.
The third polling question reveals that most companies (65%) represented in the room are not yet engaging in contextual modelling but are still doing traditional modelling.
Personal note: i would assume that the percentage that has really industrialized this is much lower.
Innovation Use Case – How to Leverage Data in the Cloud to Speed Up Decision Making and Drive Innovation
Mark Hembury, Head of Sales, Dach and CEE, NeoXam
A data centric operating model puts data at the foundation of a business. On top of a data centric foundation, a business layer can be built, which in turn serves the client and supports client centricity. Data and data storytelling will continue to grow in importance.
The data life cycle could be characterized as: acquire, normalize, validate, consolidate, enrich, store, distribute...
Personal note: It strikes me that this type of representation comes back time and time again put makes it look as if the life cycle of data stops as soon as data people have "distributed" data. To me, that's really when the value chain really begins...
In the polling question, only 8% has no intention to move to the cloud.
In financial markets, innovation is a strategic asset. It drives new products, new services, new processes and new cooperative business models.
The primary challenges with ESG are that there is a requirement to fast and easy access to large volumes of data, data needs to be mingled, golden copies need to be created, ease of access needs to be ensured and analytical insights need to be provided.
Personal note: I miss the aspect of "re-integration of insights into existing processes", to enable more automated traditional processes.
In the polling, master and reference data (44%) was identified as the most immediate focus for cloud-enabled data storage and analytics.
Centralized data management and cloud are mutually extensible, not excluding each other.
Data Science Interview - How Can You Identify and Rapidly Learn from Failures When Designing and Implementing New Science and Analytics Strategies?
Angel Serrano, Data Science and Analytics Lead, Santander
Interviewed by Mike Washington, Vice President, UK Data Ecosystem, Anonos
What is the most important first step to create a data science capability? A large majority (81% in the polling) agrees that the first step is to understand the strategy of the organisation and priorities. The second element is to understand the appetite to implement AI solutions from the top. The IT landscape can be changed in function of the first two aspects.
When implementing a data science capability, it can happen organically, through a big bang, externalize the capability, etc. It is most likely the best option to NOT externalize the full capability.
In a first stage, experimentation is essential. After that, an infrastructure is really a prerequisite, as well as data engineers. Finally, the capability to deploy needs to follow. A common view on privacy and governance is equally essential.
Interestingly, the audience gives a 0% score to the idea of externalizing the data science function to an external consulting company.
A common mistake is to think that you can solve all the problems of an organization, just because you have a few data scientists. The room considers it a major mistake to create a capability without clear goals and objectives (57%). Another mistake is to NOT hire the different roles or to NOT organize a data science life cycle.
Today, the capabilities more exist in the market, as there are more education programs that return data scientists, data engineers, etc. Finding talent has become easier, despite the war for talent.